The combination of Python programming language and SQL database management can greatly enhance your data analysis capabilities. By leveraging the strengths of both, you can unlock new levels of depth and sophistication in your data work. This comprehensive guide is designed to walk you through the entire process of integrating Python with an SQL database.
The first part of the guide will focus on setting up your environment. This involves installing the necessary software and ensuring that all the components are properly configured to work together. The goal is to provide a smooth and stable platform for your data analysis tasks.
Once the environment is set up, the guide will then walk you through the process of connecting Python to an SQL database. This is a critical step, as it allows your Python scripts to interact directly with the data stored in the database.
Once the connection is established, you will be guided on how to perform SQL queries using Python. This involves writing Python scripts that send SQL commands to the database, retrieve the results, and then process them. This part of the guide will provide detailed examples and explanations to help you understand the process.
In addition to querying the database, the guide will also cover how to analyze data using Python libraries. These powerful tools can help you visualize, explore, and understand your data in ways that go far beyond simple tables and charts.
By mastering these skills, you will be able to streamline your data handling processes, perform comprehensive data analysis efficiently, and uncover valuable insights that can inform business decisions. Whether you're a data analyst, a scientist, or a curious hobbyist, these skills can greatly enhance your ability to work with and understand data.
Setting Up Your Environment
Before we delve into the main content, it's essential that we take a moment to properly set up all the necessary tools and libraries. This preparatory step will ensure we have a smooth and efficient workflow, allowing us to focus on the task at hand without getting bogged down by technical issues. The tools and libraries we are going to use will provide the framework and foundation for our work, so it's critical that we get them up and running before we proceed.
Step 1: Install Python
If you're just starting out with your programming journey and haven't yet installed Python on your system, don't worry. You can easily download and install it from the official Python website. Simply head over to the official Python website, where you will find the latest version of Python available for download. The website is user-friendly and it should be a straightforward process to download the Python installer. Once downloaded, run the installer and follow the on-screen instructions to have Python set up on your computer.
Step 2: Install SQL
When starting a new project, it's important to choose an appropriate SQL database system. Two of the most reliable options that you might consider are PostgreSQL and MySQL. Both of these systems offer robust features and support that make them ideal for a wide range of projects.
PostgreSQL is a powerful, open-source object-relational database system that uses and extends the SQL language combined with many features that safely store and scale the most complicated data workloads. MySQL, on the other hand, is the world's most popular open-source database. Despite its powerful features, MySQL is simple to set up and easy to use.
To get started with either of these systems, you will need to install the appropriate software on your computer. Thankfully, both PostgreSQL and MySQL have clear, easy-to-follow installation instructions available on their respective websites. You can access these instructions by following the links provided below:
- For PostgreSQL, visit https://www.postgresql.org/download/
- For MySQL, visit https://dev.mysql.com/downloads/installer/
Remember, the choice between PostgreSQL and MySQL will depend on the specific requirements of your project. It's important to understand the strengths and weaknesses of both systems before making your decision.
Step 3: Install Necessary Libraries
In order to proceed with the installation of the necessary Python libraries, you'll need to utilize pip
, which is a standard package manager for Python. It's a reliable tool that allows you to install and manage additional libraries and dependencies that are not distributed as part of the standard Python library. Here's how to use it:
pip install pandas sqlalchemy psycopg2-binary pymysql
Connecting Python to Your SQL Database
In order to establish a connection between Python, a high-level programming language, and an SQL database, which is crucial for data storage and retrieval, we are going to utilize SQLAlchemy. SQLAlchemy is a highly robust and powerful ORM - Object Relational Mapper. This tool provides a full suite of well known enterprise-level persistence patterns, designed for efficient and high-performance database access which is a must for modern web and application development.
Example: Connecting to a PostgreSQL Database
First, set up your database connection using SQLAlchemy.
from sqlalchemy import create_engine
# Replace with your actual database credentials
DATABASE_TYPE = 'postgresql'
DBAPI = 'psycopg2'
USER = 'your_username'
PASSWORD = 'your_password'
HOST = 'localhost'
PORT = '5432'
DATABASE = 'your_database_name'
# Create the database engine
engine = create_engine(f'{DATABASE_TYPE}+{DBAPI}://{USER}:{PASSWORD}@{HOST}:{PORT}/{DATABASE}')
The example Python script is part of the process to integrate Python with an SQL database for data analysis. In this case, the script is designed to establish a connection to a PostgreSQL database using SQLAlchemy, which is a Python SQL toolkit and Object-Relational Mapping (ORM) library.
The code begins by importing the 'create_engine' function from the 'sqlalchemy' module. This function is essential for setting up the connection to the database.
Next, the database credentials are defined. These are specific to the user's database and must be replaced with the actual credentials for the database you intend to connect to. The defined credentials include the type of database (in this case, PostgreSQL), the DBAPI ('psycopg2' which is a PostgreSQL adapter for Python), the username and password for the database, the host (which is typically 'localhost' if the database is hosted on the same machine where this script is running), the port number (5432 is the default port for PostgreSQL), and the name of the database.
Then, the 'create_engine' function is invoked to create the database engine. The engine is a common interface to the database from SQLAlchemy and it's created by providing the connection URL. The connection URL follows the format:
engine = create_engine('postgresql+psycopg2://user:password@localhost:5432/mydatabase')
In the script, the connection URL is built using an f-string for easier readability and modification. The f-string includes placeholders for the various components of the URL, which are filled in with the previously defined variables.
The resulting engine object represents the core interface to the database. It provides a source of connectivity to the database and allows the execution of SQL queries and commands.
Understanding how to create a database connection is a crucial part of integrating Python and SQL for data analysis. Once the connection is established, Python scripts can interact directly with the data stored in the database, allowing for efficient and sophisticated data analysis tasks.
Example: Connecting to a MySQL Database
If you are using MySQL, the connection setup would look like this:
from sqlalchemy import create_engine
# Replace with your actual database credentials
DATABASE_TYPE = 'mysql'
DBAPI = 'pymysql'
USER = 'your_username'
PASSWORD = 'your_password'
HOST = 'localhost'
PORT = '3306'
DATABASE = 'your_database_name'
# Create the database engine
engine = create_engine(f'{DATABASE_TYPE}+{DBAPI}://{USER}:{PASSWORD}@{HOST}:{PORT}/{DATABASE}')
This block of Python code establishes a connection to a MySQL database using SQLAlchemy, a powerful SQL toolkit and Object-Relational Mapping (ORM) system in Python.
The script begins by importing the 'create_engine' function from the 'sqlalchemy' module. The 'create_engine' function is a core component of SQLAlchemy, which establishes a source of connectivity to the database server.
Next, the script outlines a series of constant values, each representing a piece of information necessary to connect to the database. These constants include 'DATABASE_TYPE', 'DBAPI', 'USER', 'PASSWORD', 'HOST', 'PORT', and 'DATABASE'.
- 'DATABASE_TYPE' is set to 'mysql', indicating the type of database to which the script is connecting.
- 'DBAPI' is set to 'pymysql', specifying the database API (Application Programming Interface) that Python uses to communicate with the MySQL database.
- 'USER' and 'PASSWORD' are placeholders for your actual MySQL username and password.
- 'HOST' is set to 'localhost', meaning the database server is hosted on the local machine. If your database server is hosted elsewhere, you would replace 'localhost' with the relevant IP address or server domain name.
- 'PORT' is set to '3306', which is the default port for MySQL servers. If your server runs on a different port, you would replace '3306' with the appropriate port number.
- 'DATABASE' is a placeholder for the actual name of the MySQL database to which you're connecting.
The final part of the script calls the 'create_engine' function and passes a formatted string that contains all the necessary information to establish a connection to the database. This function returns an instance of an 'Engine' that represents the core interface to the database, which is capable of executing language-agnostic SQL statements and commands.
This script is a fundamental step in integrating Python with a MySQL database for purposes such as data analysis or web backend development. Once the connection is successfully established, you can use Python to interact with the MySQL database, allowing you to perform operations like querying data, inserting new data, updating existing data, or deleting data.
Performing SQL Queries with Python
Once you have successfully established a connection, you can then begin to utilize SQLAlchemy's capabilities. SQLAlchemy is a SQL toolkit that gives application developers the full power and flexibility of SQL. It provides a full suite of well-known enterprise-level persistence patterns, designed for efficient and high-performing database access. You can use it to execute SQL queries, which is a powerful feature that allows you to interact with your database in a more efficient and secure manner.
Example: Executing a SELECT Query
import pandas as pd
# Write a SQL query
query = "SELECT * FROM your_table_name"
# Execute the query and load the data into a pandas DataFrame
data = pd.read_sql(query, engine)
print(data.head())
This Python script uses pandas, a data manipulation library, to execute a SQL query and load the result into a DataFrame, which is a two-dimensional labeled data structure.
Here, 'import pandas as pd' loads the pandas library. The 'query' variable stores a SQL query, which is meant to select all data from a table ('your_table_name' should be replaced with the actual table name). 'pd.read_sql(query, engine)' executes the SQL query using a connection engine and loads the data into a pandas DataFrame. The 'print(data.head())' statement prints the first five rows of the DataFrame.
Example: Executing an INSERT Query
# Write an SQL insert statement
insert_query = """
INSERT INTO your_table_name (column1, column2)
VALUES ('value1', 'value2')
"""
# Execute the insert query
with engine.connect() as connection:
connection.execute(insert_query)
This Python script contains an SQL query to insert data into a database. It first defines an SQL insert query that will add a row of data ('value1', 'value2') into 'your_table_name' under columns 'column1' and 'column2'.
This query is stored in the variable 'insert_query'. Then, using a connection to the database established through 'engine.connect()', the script executes the 'insert_query'. The 'with' statement ensures that the connection is properly closed after the query is executed.
Analyzing Data with Python
Pandas is an open-source, BSD-licensed Python library providing high-performance, easy-to-use data structures and data analysis tools. With the help of pandas, you are able to manipulate and analyze data in a more efficient way. This is particularly useful when dealing with data that has been retrieved from a SQL database. You can perform a variety of operations, such as cleaning the data, transforming it, and analyzing it to extract valuable insights. This makes pandas a vital tool for any data scientist or analyst.
Example: Data Cleaning
# Load data from the database
data = pd.read_sql(query, engine)
# Drop rows with missing values
cleaned_data = data.dropna()
# Display cleaned data
print(cleaned_data.head())
This is a Python code snippet that does the following:
- It loads data from a database using a SQL query. The 'pd.read_sql' function is from the pandas library and it executes the SQL query on the specified database engine.
- It removes any rows in the data that have missing values using the 'dropna()' function. This function returns a new DataFrame with the rows dropped.
- Finally, it prints the first five rows of the cleaned data using the 'head()' function. This is typically used to preview the data.
Example: Data Aggregation
# Load data from the database
data = pd.read_sql(query, engine)
# Group by a specific column and calculate the mean
aggregated_data = data.groupby('column_name').mean()
# Display aggregated data
print(aggregated_data)
The example Python code does the following:
- It loads data from a database using a SQL query and a pre-existing engine connection, storing the result into a variable named 'data'.
- It groups the loaded data by a specific column ('column_name') and calculates the mean (average) of each group. The result is stored in the 'aggregated_data' variable.
- Finally, it prints the aggregated data to the console.
Example: Data Visualization
To visualize data, you can use libraries like Matplotlib or Seaborn.
import matplotlib.pyplot as plt
import seaborn as sns
# Load data from the database
data = pd.read_sql(query, engine)
# Create a simple plot
sns.set(style="darkgrid")
plt.figure(figsize=(10, 6))
sns.countplot(x='column_name', data=data)
plt.title('Count of Column Name')
plt.xlabel('Column Name')
plt.ylabel('Count')
plt.show()
This is a Python script that imports the matplotlib and seaborn libraries for data visualization. It then loads data from a database using a SQL query and stores it in the 'data' variable. It sets a darkgrid style for the seaborn plots. It then creates a figure of size 10x6, and generates a count plot (a type of bar plot) for a specified column in the data. The x-axis represents the unique values in the column and the y-axis represents the count of each unique value. The title of the plot is 'Count of Column Name', and the x and y axes are labeled 'Column Name' and 'Count' respectively. The plot is then displayed.
Conclusion
The integration of Python and SQL presents an incredibly powerful toolset for the analysis of data. These two technologies, when combined, let you tap into their individual strengths, creating a robust environment for dissecting and analyzing information. The first step is to set up your environment, which will serve as the base where you can seamlessly blend Python and SQL. Next, you will establish a connection with your database, a vital step that enables the interactive exploration of your datasets.
Once connected, you can start performing SQL queries. The ability to query your data using SQL is a fundamental skill in data analysis that allows you to retrieve specific data from your database. After retrieving your data, Python steps in as a versatile tool for data manipulation. With Python, you can clean and transform your data, making it easier to work with and analyze.
Beyond data manipulation, Python is also an excellent tool for data visualization. By translating your data into visual formats like charts or graphs, you can more easily identify patterns and trends, leading to deeper and more insightful analysis.
For those interested in delving deeper into the synergistic use of Python and SQL for data analysis, we recommend our comprehensive guidebook titled "Python and SQL Bible." This book offers a thorough exploration of more advanced techniques, providing a detailed roadmap for those seeking to maximize their data analysis capabilities.
FAQs
What are the benefits of integrating Python and SQL?
Integrating Python and SQL allows you to efficiently handle large datasets, perform complex queries, and leverage Python's powerful data manipulation and analysis libraries.
How do I connect Python to an SQL database?
You can connect Python to an SQL database using SQLAlchemy, a powerful ORM. It supports various database systems, including PostgreSQL and MySQL.
What libraries do I need to integrate Python and SQL?
You need libraries like pandas
for data manipulation, SQLAlchemy
for database connection, and specific database drivers like psycopg2-binary
for PostgreSQL or pymysql
for MySQL.
How can I perform data analysis with Python?
Using libraries like pandas
for data manipulation, Matplotlib
and Seaborn
for data visualization, you can perform comprehensive data analysis with Python.
Where can I learn more about integrating Python and SQL?
For more detailed information and advanced techniques, check out our book "Python and SQL Bible."
Discover "Python and SQL Bible”
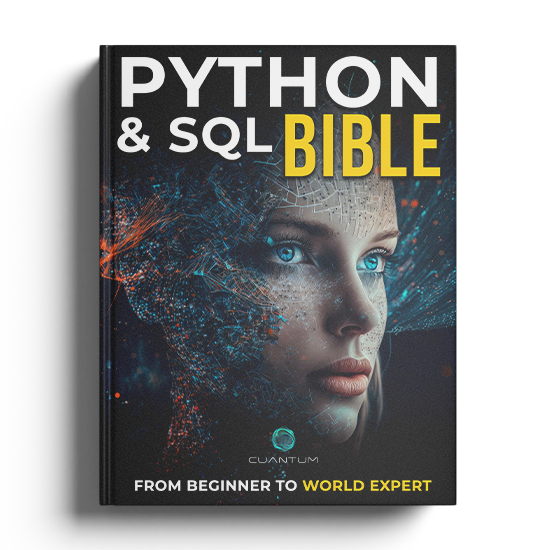
Why Choose This Book?
- Comprehensive Coverage: Covers everything from the basics to advanced techniques, providing a thorough understanding of Python and SQL integration.
- Practical Examples: Includes real-world examples and practical exercises to help you apply what you've learned.
- Detailed Explanations: Breaks down complex topics into easy-to-understand sections, making it accessible for all skill levels.
- Hands-On Exercises: Engage in hands-on exercises at the end of each chapter to reinforce your learning and build confidence.
- Structured Learning Path: Follows a structured learning path that gradually builds your knowledge and skills.
- Automation Techniques: Learn how to automate database operations, reducing manual workload and improving efficiency.
Don't miss out on the opportunity to master Python and SQL. Get your copy of "Python and SQL Bible" today and start transforming your data management and analysis processes!